Moving Away from ‘Average,’ Toward the Individual
In a course from Prof. David Lydon-Staley, seven graduate students applied an “n of 1” methodology to better capture the nuances of a diverse population.
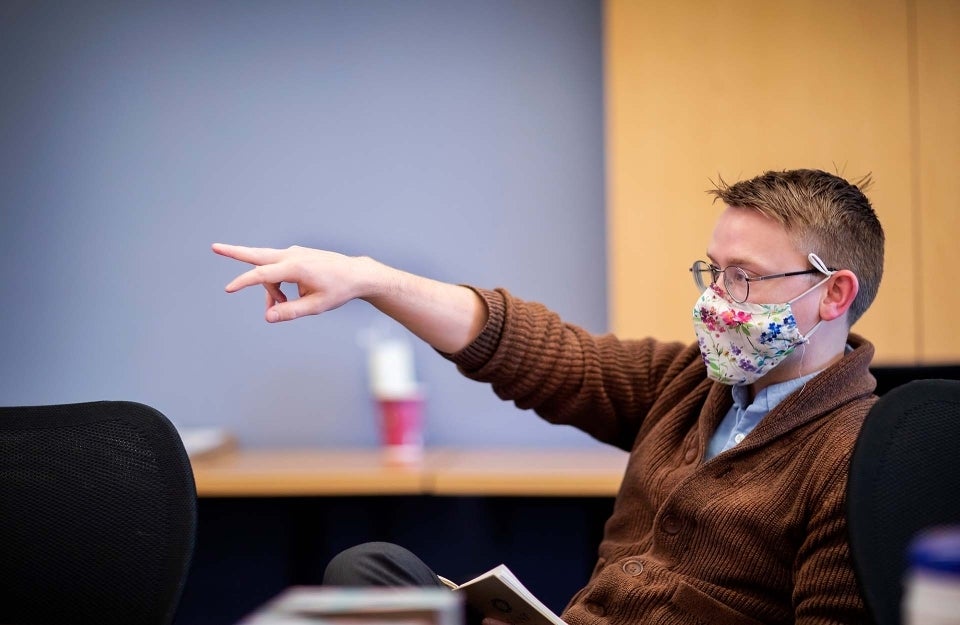
David Lydon-Staley is an assistant professor of communication and principal investigator of the Addiction, Health, & Adolescence Lab at the Annenberg School for Communication.
- Randomized control trials look at the average of large groups of participants, but often fail to capture the experience of women and minoritized racial and ethnic groups.
- David Lydon-Staley’s grad students used an “n-of-1” approach to conduct an experiment on themselves to gain an understanding of experimentation on the individual level.
- Lydon-Staley proposes that by scaling up from individual level findings, we might find patterns that cluster naturally, rather than artificially.
To prep for an upcoming course he was teaching, professor David Lydon-Staley decided to conduct an experiment: Might melatonin gummies—supplements touted to improve sleep—help him, as an individual, fall asleep faster?
For two weeks, he took two gummies on intervention nights and none on control nights. The point, however, wasn’t really to find out whether the gummies worked for him (which they didn’t), but rather to see how an experiment with a single participant played out, what’s known as an “n of 1.”
Randomized control experiments typically include hundreds or thousands of participants. Their aim is to show, on average, how the intervention being studied affects people in the treatment group. But often “there’s a failure to include women and members of minoritized racial and ethnic groups in those clinical trials,” says Lydon-Staley, an assistant professor at the Annenberg School for Communication. “The single-case approach says, instead of randomizing a lot of people, we’re going to take one person at a time and measure them intensively.”
In Lydon-Staley’s spring semester class, Diversity and the End of Average, seven graduate students conducted their own n-of-1 experiments—on themselves—testing whether dynamic stretching might improve basketball performance or whether yoga might decrease stress. One wanted to understand the effect of journaling on emotional clarity. They also learned about representation in science, plus which analytical approaches might best capture the nuance of a diverse population and individuals with many intersecting identities.
“It’s not just an ‘n of 1’ trying to do what the big studies are doing. It’s a different perspective,” says Lydon-Staley. “Though it’s just one person, you’re getting a much more thorough characterization of how they’re changing from moment to moment.”
Individual n-of-1 experiments
In a small classroom on the third floor of the Annenberg School for Communication, second-year doctoral student Adetobi Moses kicked off the trio of presentations happening that mid-April day. “My intent for the study was journaling,” she says.
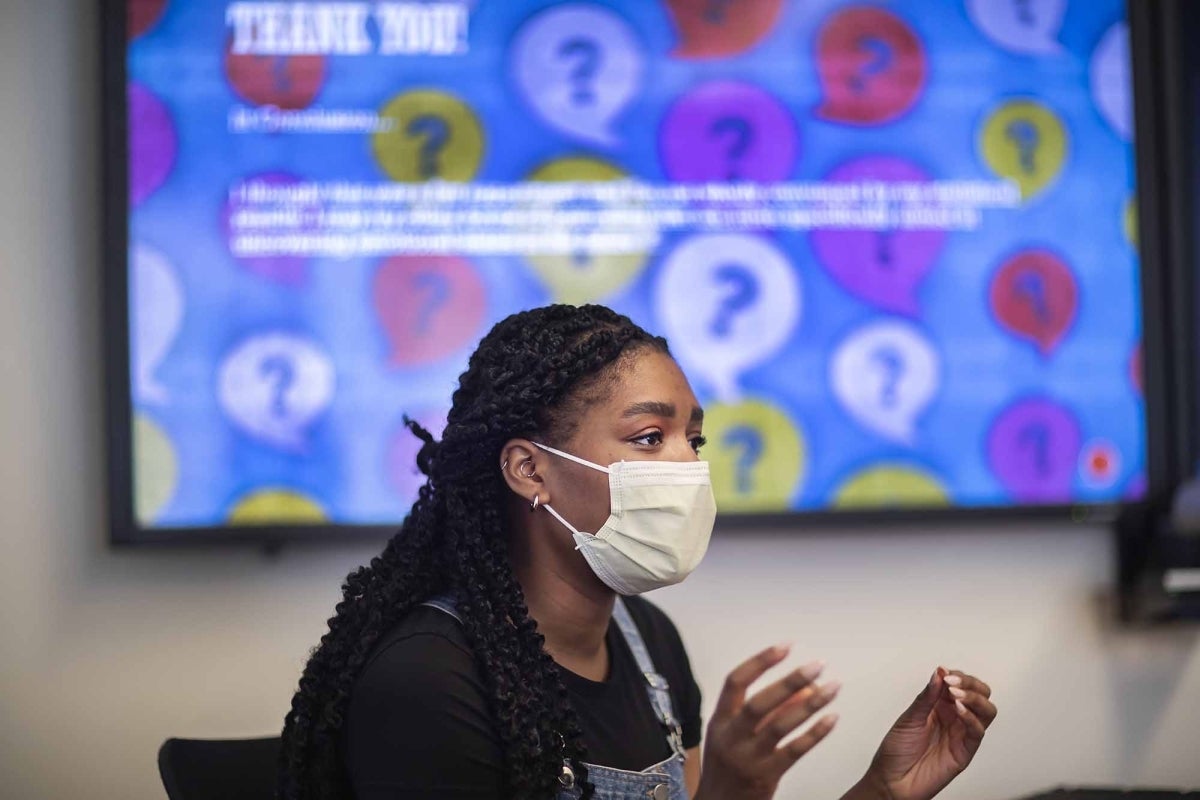
She described the different options, including her choice of stream-of-consciousness journaling, then talked through her two-week experiment. In the end, her data showed that the writing helped only minimally with her emotional clarity. But the process itself? She found it empowering, a sentiment that others in the room echoed. Despite experimental results that may have lacked statistical significance, the grad students appreciated gaining deeper insight into an aspect of themselves.
Sometimes the results surprised them, too, like those of Darin Johnson, a third-year Ph.D. student studying code-switching. For his experiment, he wanted to understand whether reducing social media use on his phone would drop his stress level. “I follow a lot of social justice–oriented pages, which include a lot about racism and police brutality. I would just sit there scrolling and be stressed out,” he says. He thought removing the input that caused these reactions might prevent the anxiety associated with them.
So, on intervention days, he would receive a notification when he reached the time limit that he’d set. On control days, his access remained unlimited. At the end of each day, he took a survey that he’d created. Before even analyzing his data, he realized that avoiding social media didn’t actually help him but instead made him feel isolated, cut off from his circle. “It’s counterintuitive,” he says.
Applying the framework broadly
The notion of n-of-1 experiments often raises eyebrows, says Lydon-Staley. “I’m on the fringe here, but I think it’s the way to go,” he says. “Randomized control trials give you a statistic for the ‘average person,’ but that’s a statistical artifact that doesn’t exist. I want to know what works for me.”
Lydon-Staley applies this framework to much of the research conducted in his Addiction, Health, & Adolescence Lab. For example, in a project about smoking cessation, he and his team are collecting reports from participants about their specific withdrawal symptoms—cravings, irritability—10 times a day for 10 days, before and during a quit attempt. In a project about alcohol and the brain, participants get brain scans five times sober, five times after drinking alcohol.
“We say, ‘On average, when people drink, the brain looks like this,’ but we’re making a huge assumption that the average we’re getting can tell us about the individual,” Lydon-Staley says. “I don’t think that’s the case. Literally everything I do has been collecting lots and lots of data on individuals to get at our heterogeneity. This is pushing us to gain a better understanding at the individual level.”
"Though it’s just one person, you’re getting a much more thorough characterization of how they’re changing from moment to moment." —David Lydon-Staley
Personalized medicine has already moved in this direction, using genetics and other biomarkers to guide treatment. “So many people deal with medical issues that may not have a one-size-fits-all solution,” says second-year Ph.D. student Baird Howland, who is also in the class. “Anybody could, in theory, do this type of experiment to figure out what works and what doesn’t for them.”
Lydon-Staley sees great potential in the ability to scale up the single-case approach: Collect enough samples and patterns will emerge revealing natural rather than artificial clusters. “Often, you can’t take an intersectional approach with statistics,” Johnson says. “People might aggregate by race or by gender. Those are disparate categories, but I’m gay and Black. If I were to do a statistical analysis, I’d have to separate them out, and ‘n of 1’ allows us not to.”
‘The full person’
The point of the class isn’t just to show these graduate students a different approach to science but also to get them thinking about the advantages of research that includes broad representation. “The people we recruit into our studies benefit the most from the science,” says Lydon-Staley. “The findings are more applicable to them than people who aren’t collected as part of the data.”
That said, Lydon-Staley isn’t suggesting throwing out the baby with the bathwater, as he puts it. “I’m biased, and I recognize that. This type of methodology is not something we necessarily learn even though it’s intuitive,” he says. “You need a course like this to dive into the methods, which can hopefully make it easier to collect a more diverse sample.”
Johnson and his classmates have become quick converts. “I like the fact that you can focus on the full person,” he says.
Even Howland, who studied physics as an undergrad and is now looking at the effect of mainstream news on public agendas for his doctoral work, sees how this approach can succeed. “My future work doesn’t map super well to these individual-based treatments, but I wouldn’t rule it out,” he says. “It’s a nice reminder that you can think about your individual issues scientifically and learn something about yourself. It’s something that could catch on.”
Lydon-Staley’s seen it happening more already. But, he says, even if the methodology stays on the perimeter for now, he’s grateful for the chance to get students thinking about new ways to make science more diverse and representative. “No one is just one thing. They’re also a specific race, a specific ethnicity, a specific sexual orientation. It’s hard to think about how to capture those,” he says. “With the bottom-up approach, you start at the person level.”